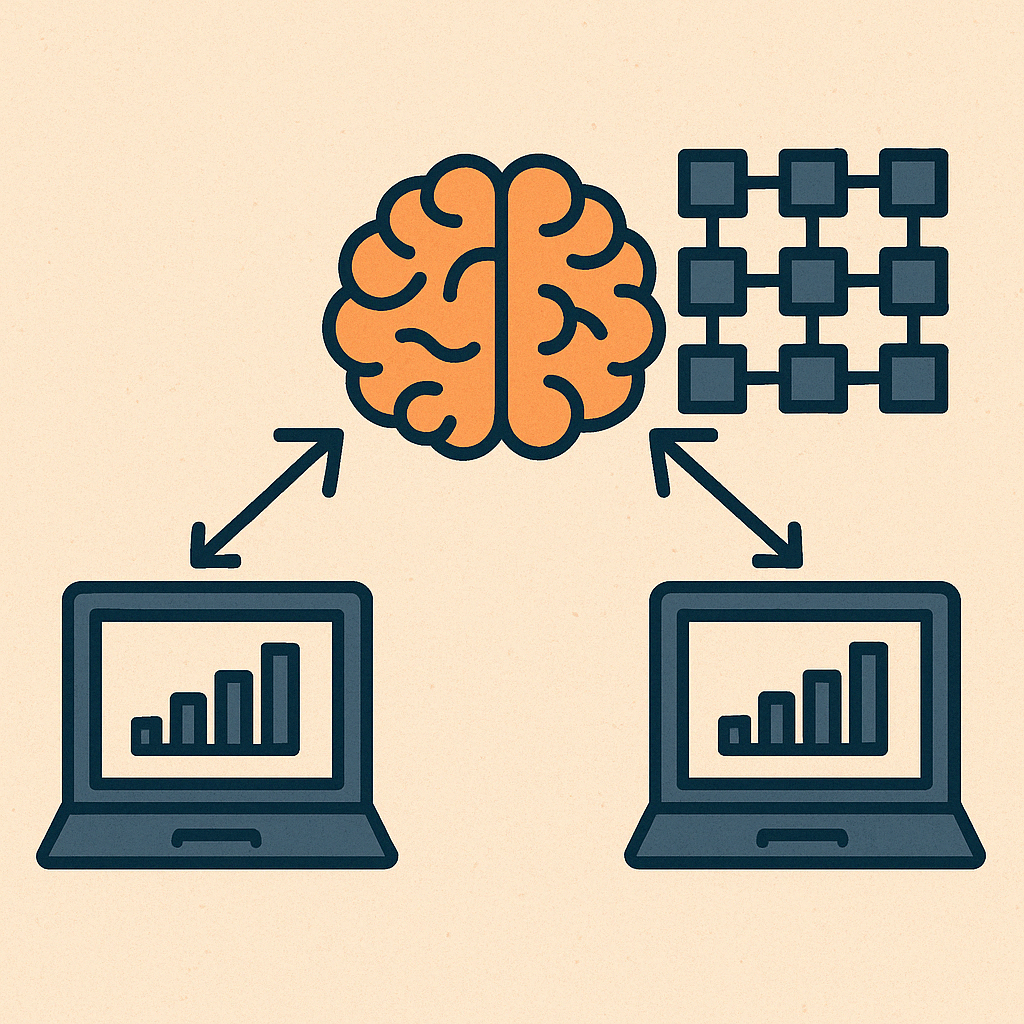
By Ghazaleh Keshavarzkalhori, Cristina Perez-Sola, Guillermo Navarro-Arribas, Jordi Herrera-Joancomartí, and Habib Yajam
In a world where data privacy is more important than ever, federated learning has emerged as a powerful way to train machine learning models collaboratively—without ever needing to share raw data. Originally introduced by Google, this approach allows data owners to train models locally on their data, and then send those trained models (not the data) back to a central server where they are aggregated into a global model.
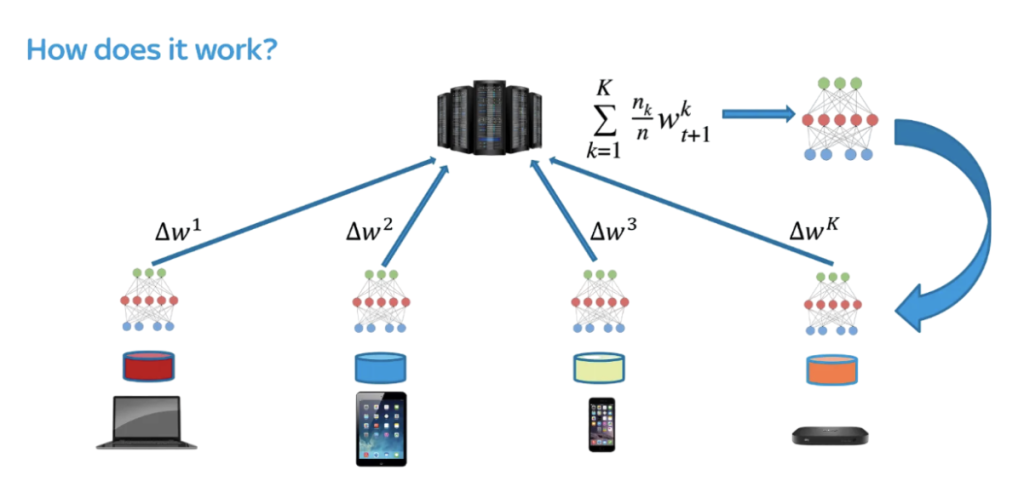
It’s an elegant solution—until you start looking at the privacy and security challenges under the hood.
Why Federated Learning Isn’t Enough for Privacy?
Federated learning was initially praised for its privacy benefits. For instance, Google used it to train the Gboard keyboard while keeping users’ texts private. Let’s think about it intuitively. If we all send our models to a server to be combined, someone could try typing in half-finished sentences and see how our model responds. That could give them some information on how we usually write—which is a big privacy issue.
Assuming the model is well-trained, the output of the model would be what I will probably say. This means that anyone with a predefined dictionary of sentences could infer how I might write or complete a sentence.
In more general and technical terms, three different attacks explained below can be applied to a simple federated learning:
- Membership inference attacks can detect whether a specific user’s data was used to train the model.
- Property inference attacks can guess hidden details like geographic location or device environment.
- Reconstruction attacks can even attempt to recreate the original data itself.
All these refer to the privacy of the training, analyzing how much information can be leaked when training is distributed among multiple data owners, instead of a central server. However, with distribution always comes the question of trust. How much can we trust the training if it’s not done by our device and by a group of data owners?
Unfortunately, the answer is no—we cannot fully trust the process.
In the federated learning setting, the trust is defined in two different concepts. Let’s think of an enterprise scenario where multiple competitors, companies providing anomaly detectors for networks, gather to train a general detector together. This scenario makes sense because mostly network data is private to the companies and there are considered confidential and are not shared easily, however the information from a simple network is not nearly enough to detect anomalies happening in different networks so generalization of the model is a must.
In our scenario, we can easily understand the security risks imposed by the federated learning asking the two following questions.
- Can we trust other companies wouldn’t generated false data to mess up the model and get ahead of their competitors?
- Can we trust that the server bundling all our models is correctly computing the final model? Can we trust that our models are fairly treated in the sever?
The first question is referred to as poisoning, and the second one as unfair server behavior.
Introducing Federify: A New Approach
To address these issues, we propose Federify, a verifiable federated learning scheme that combines zkSNARKs, blockchain, and homomorphic encryption. A proof-of-concept implementation of Federify is also available on GitHub: FederatedNaiveBayesZKP.
Let’s see how we deal with each of the problems.
Unfair central server →
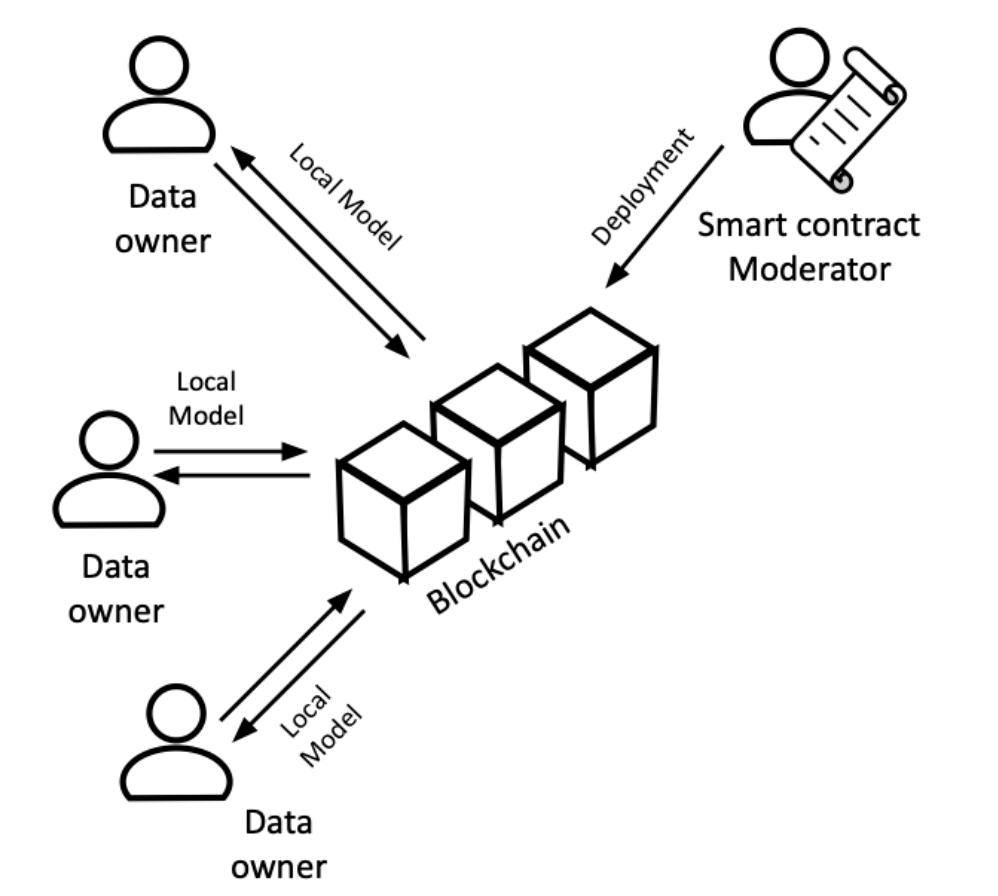
Instead of relying on a traditional server to bundle our models, we can use blockchain and smart contracts for better transparency. Smart contracts are self-executing programs on the blockchain. Their transparency and automation allow for verifiable model aggregation, eliminating the need to trust a central server. This way the computation of the global model can be done in a trustless manner.

Untrusted Data owners →
Zero-knowledge proofs are probabilistic mathematical proofs that prove a statement without revealing extra information about it. Sounds complicated, right?
If you’re interested in ZKPs and more specifically zkSNARKs, I encourage you to start your journey learning about them here.
For the sake of this post, we will assume that ZKPs are toolsets which when passed arbitrary computations and some inputs, they can prove that the output of that computation with the specified inputs is a certain value. Basically binding the computation to its output. In addition to this binding, it also can hide specific inputs to the computation providing us with privacy.
In Federify, we handle the problem of untrusted data owners with the help of zkSNARKs. Each data owner trains their model on their local data and generate proof for the training sending the model and the proof to the smart contract. The smart contract will only join any local model with the global one if it’s able to verify the correctness of the model training.
Information leakage of local data through models →
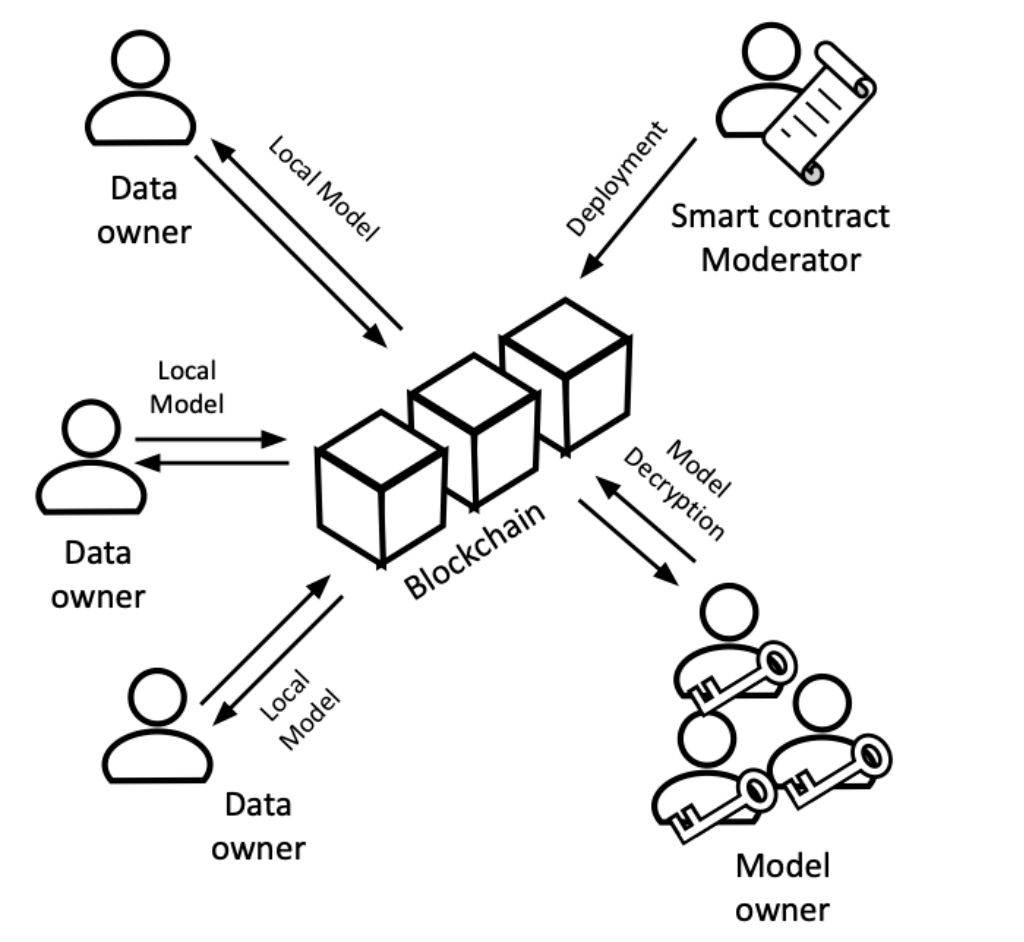
This problem rises from publicly sharing our local models in plaintext. Let’s go back to the intuitive attack we thought of at the start. If anyone on the blockchain can see the model I’m sending to the smart contract, either one of them can use their crafted inputs on my model and extract information. For hiding our models we can just easily hide them. This can be done in multiple manners, but most of them require unhiding the models before doing any computations on them. Since the computations are done by the smart contract and publicly this indicates no hiding at all. To tackle this problem, we hide our models using homomorphic encryption. With homomorphic encryption, data can be processed without decrypting it. Federify uses a multi-party version of this, meaning no single participant can decrypt models—they must collaborate to unlock any information.

In conclusion Federify handles the security and privacy risks in a federated learning structure as below:
- Using smart contracts with transparent computations to handle with unfair servers.
- Using homomorphic multi-party computations to hide the models and preserver privacy.
- Using zkSNARKs to prove computations carried out locally and remove trust from the training.
For further technical details on this scheme, please read our paper:
Federify: A Verifiable Federated Learning Scheme Based on zkSNARKs and Blockchain